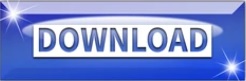
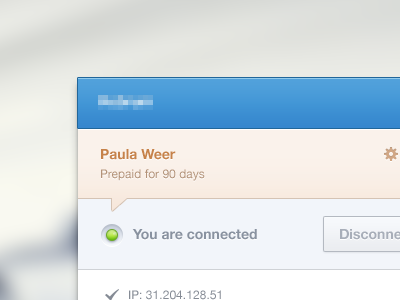
Sun Seeker - Finding a Window's Sunlight Exposure.Sun Seeker - Seeing the Light with Augmented Reality.Samples of Fonts Available on the iPhone.
Ifart sales mac#
Indie Mac and iOS Developer Influence Rankings.Everywhere – Timezones, Holidays and Current Weather.Comparing Satellite Image Providers in iOS and OSX.Sun Seeker – Finding a Window’s Sunlight Exposure.Next Next post: App Store Icon Rendering Change Search for: Search Recent Posts As more data becomes available I will add it in to the mix, to see how much further this can be refined. So there you have it – the best that I can do with the available data. I therefore suspect that the US curve power factor is more realistic for the long tail, and that the drop-off in sales levels for Australia well below the #20 ranking might be somewhat less than that predicted by the given equation. the power factor to be the same in each equation). However, note that the Australian curve is based on data only going down to #17 ranking, whereas the US curve has data down to #100 ranking. Given that there is no reason to suspect any major difference in sales dynamics, one might have expected the ratio to remain similar at all rankings (ie. In fact, for the given equations, the World/Australia sales ratio varies from 35 (#1 ranking) to 13 (#50 ranking). It roughly confirms my earlier guess that world sales were typically 30 times more than Australian sales. This equation can probably be used to give a reasonable estimate of sales of paid apps in the US iTunes app store using the overall ranking. I suspect that sales at the #1 spot will be much more variable than lower ranked slots, so it seemed sensible to include only the lowest figures that resulted in a #1 ranking, for this purpose.Īs before I tried fitting logarithmic, exponential and power curves to the data – and again the power curve gave by far the best fit, with the approximate equation


Also note that, to clarify the picture, I did exclude some of the higher figures given for #1 ranking – up to 50,000 or more supposedly. The main assumption here is that US rankings reflect Worldwide rankings reasonably well, which although not necessarily true for specific apps, is probably true on average. Using data from these sources – some real sales figures and others inferred and estimated, I constructed the following graph. We now have a good idea of the apponomics of the Australian store, but what about world-wide sales? To begin to answer this, I have gathered as much data as I could find giving US store app ranking versus daily sales. For example while Oz Weather was ranked #1 sales varied between about 260 and 620, and at rank #2 from 190 to 420. However note that there is a wide range of variation about this mean trend line on a day to day basis. This equation can be used to give a reasonable estimate of sales of paid apps in the Australian iTunes app store using the overall ranking (not the ranking in any individual category such as entertainment, lifestyle, weather etc). I tried fitting logarithmic, exponential and power curves to the data – the power curve gave by far the best fit, with the approximate equation The graph below shows the entire history of sales, minus that week’s worth of “anomalous” sales data.
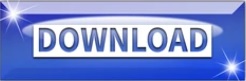